TrAgS
TrAgS - DFG Individual Grant (2023-2025)
Tracking the use and adoption of agricultural technologies through satellite remote sensing and self-supervised deep learning
Background
Novel technologies and farm management systems are considered to be crucial to increase agriculture’s sustainability and resource use efficiency. However, our data sources that provide information on what technologies are actually being used on farms are very limited. This makes it difficult to assess if technologies can indeed realize their promised environmental effects and also limits our possibilities to study and understand technology diffusion processes.
Hypothesis and Aims
Satellite data and novel deep learning approaches offer currently unused opportunities that can help to overcome those data limitations. So far, however, those datasets are not fully exploited to study adoption behavior or diffusion processes. Further, although the existing data products offer substantial opportunities, they remain limited in terms of the regions/technologies they cover. Extending to other regions/technologies is limited because learning new machine learning models or extending existing models requires large amounts of labeled reference data (i.e., observations with known reference information) that are often lacking. The aims of this project are therefore twofold.
1) First, we aim to exploit data products derived from satellite data to study diffusion processes, particularly adoption (disadoption) dynamics and spatially mediated peer effects.
2) Secondly, we aim to significantly reduce the number of needed labeled reference data for deep learning approaches when extending the approach to other regions/technologies.
Approach
We exploit an existing large scale dataset for low tillage in the US derived from satellite data to study diffusion processes. Additionally, we intend to develop novel learning procedures, and we specifically explore if the inclusion of agronomic domain knowledge can help to reduce the required number of labeled reference data. We benchmark the developed approaches against existing US data and apply them to create new data products covering other regions (Germany) and technologies (low tillage and glyphosate usage). The derived dataset will be made available publicly. We will use it to derive diffusion dynamics for low tillage and glyphosate usage in Germany which can deliver an important contribution for the ongoing policy debate around those technologies.
Group Contribution
Hugo Storm (PI), together with Ribana Roscher
PhD Student
Alexa Leyens
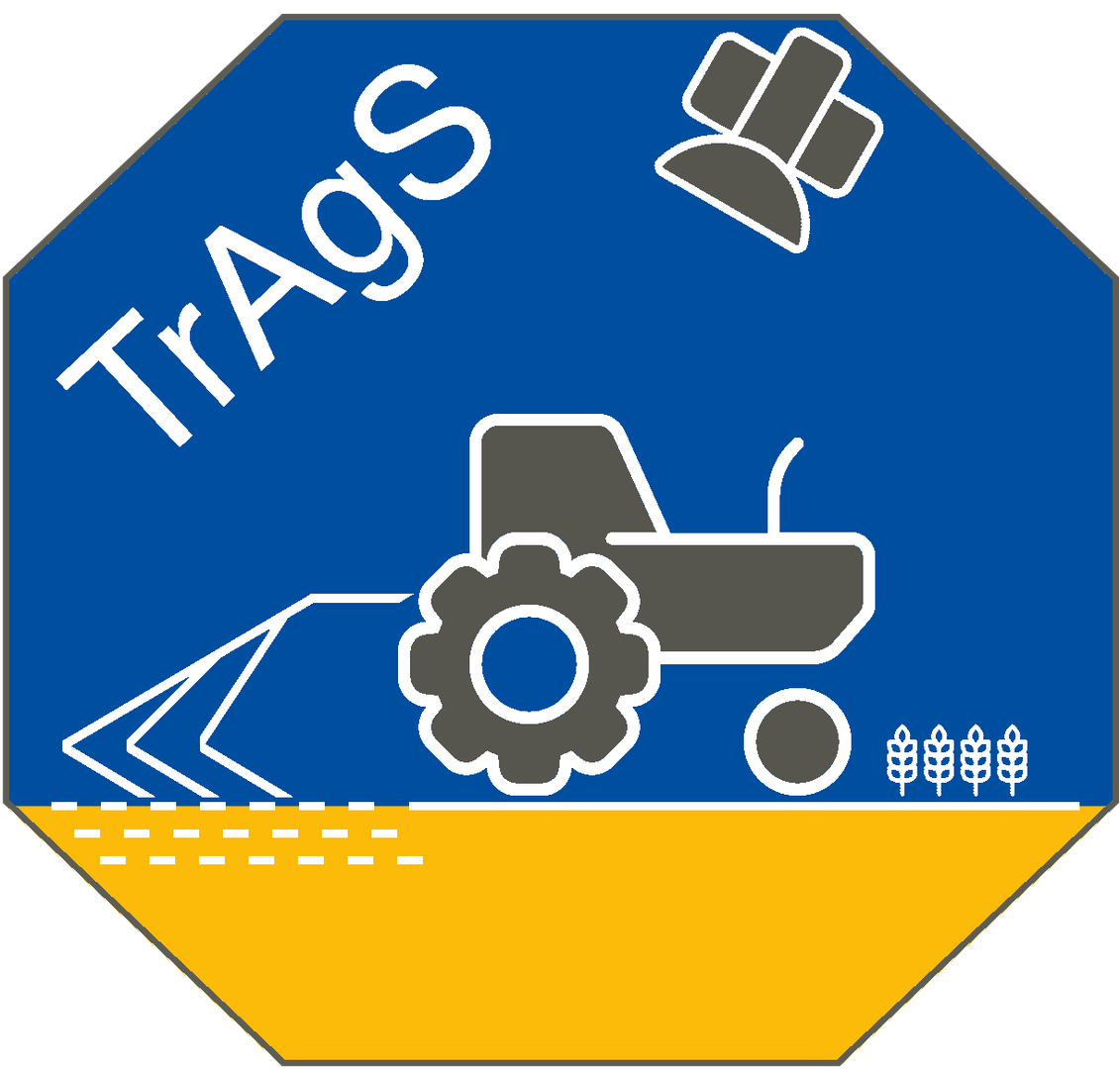